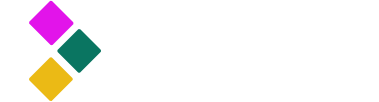
Finding the Best Claude Model for a Legal Use Case
Dataster helps you build Generative AI applications with better accuracy and lower latency.Introduction
Legal Tech companies are increasingly turning to Generative AI models to enhance their software solutions. The Claude model family, developed by Anthropic, has emerged as a popular choice for the legal vertical. With its advanced capabilities and focus on safety, Claude is well-suited for various legal applications. However, there are several models in this family, each offering different performance and price points. In this article, we will explore the different Claude models available on Dataster and help you determine which one is best suited for your legal use case
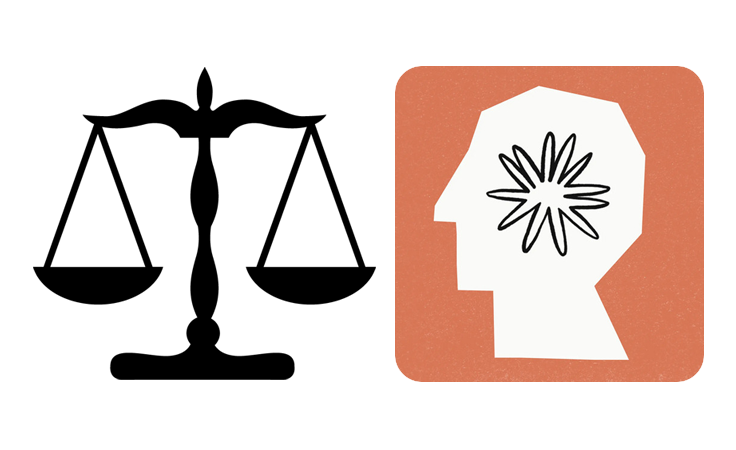
Use Case
In this use case, we want to build a legal assistant that can help lawyers draft contracts, review legal documents, and provide legal advice. The assistant should be able to understand complex legal language and provide accurate and relevant responses to user queries. We will evaluate the performance of different Claude models in this context.
We built a use case file that contains 20 user queries related to constitutional law. The queries are designed to test the model's ability to understand legal language and provide accurate responses. To allow for automated evaluation, we also provided for each query, the ground truth that contains the expected responses. The file can be found here and we are going to use the bulk upload feature to add it to our prompt catalog. This will allow us to easily evaluate the performance of different models on the same set of queries. Bulk upload instructions can be found here. In addition, the file contains a simple system prompt that instructs the model to act as a legal assistant.
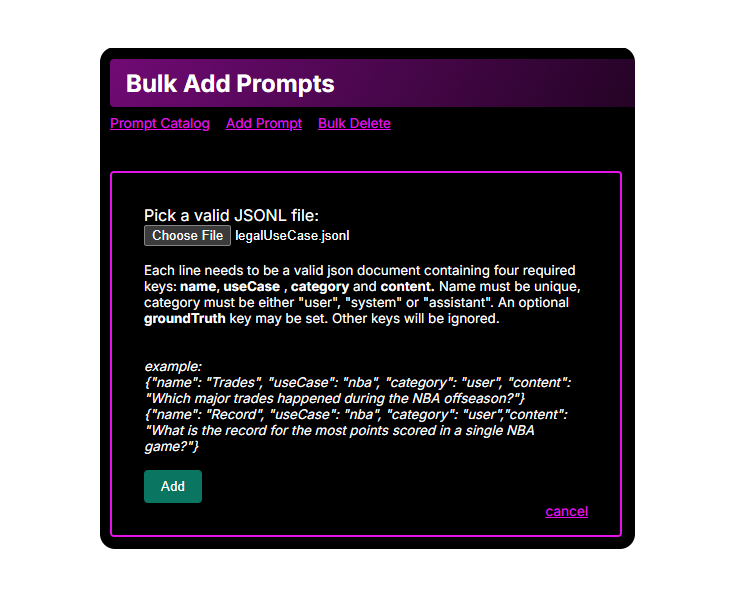
Once the file has been processed, the system and user prompts are available in the prompt catalog.
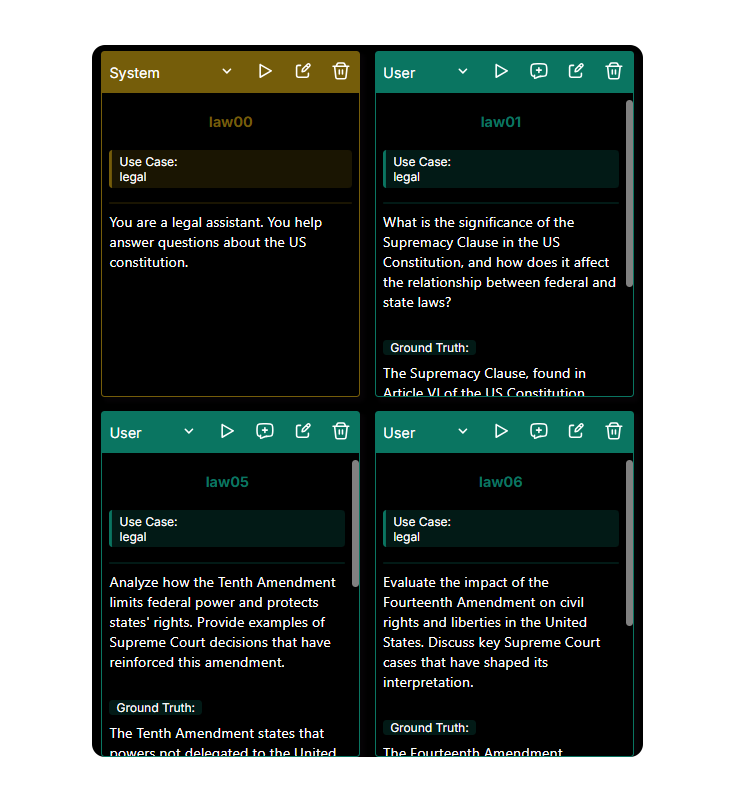
Models
At the time of writing, there are 4 Claude models available on Dataster:
- Claude 3 Sonnet
- Claude 3 Haiku
- Claude 3.5 Haiku
- Claude 3.7 Sonnet
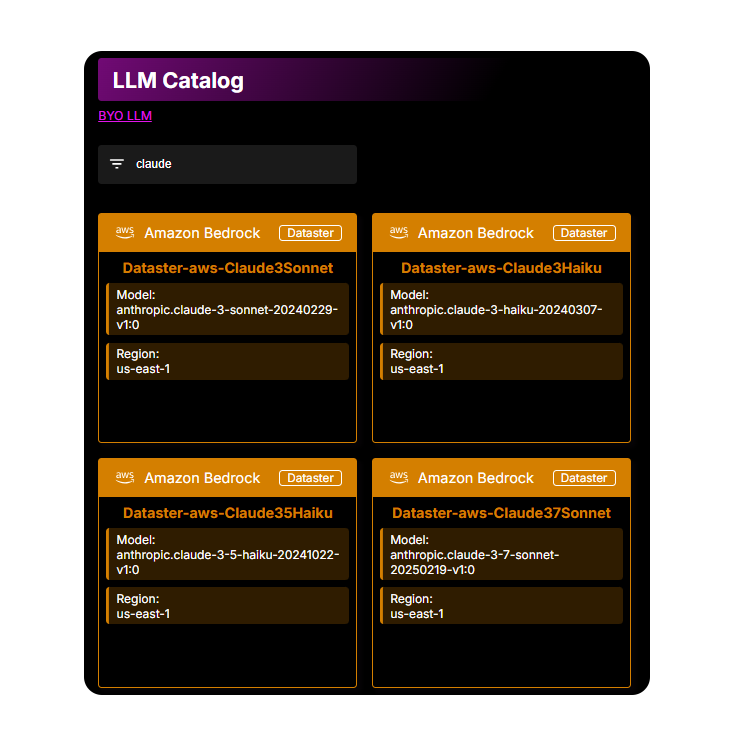
Latency
We want our legal assistant to be fast and responsive, so we will evaluate the latency of each model using the Latency Test feature. This feature allows us to measure the time it takes for each model to respond to a set of queries. It returns the average latency across all the queries in the use case as well as the standard deviation. We will run the test on the same set of queries that we used to build the use case file and compare the results. We have 1 system prompt and 20 user prompts in the use case file that we want to systematically run against 4 models. So Dataster will run 80 tests in total
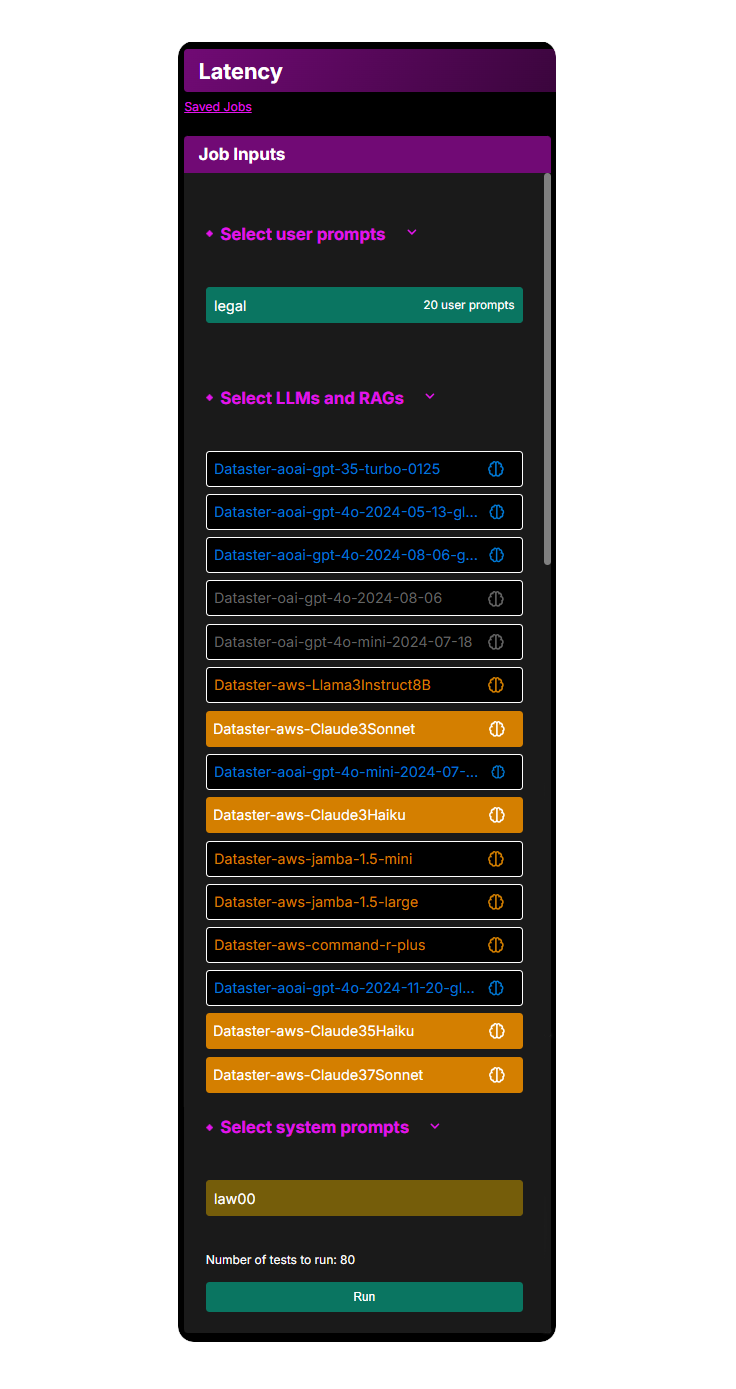
The tests reveal some drastic differences in latency between the models and underscore the importance of choosing the right model for your use case. Claude 3 Haiku is the fastest model, with an average latency of 6.98 seconds per query. It is also the most predictable with the lowest standard deviation of 1.16 seconds. At the opposite end of the spectrum, Claude 3 Sonnet is the slowest model, with an average latency of 20.52 seconds per query and the largest standard deviation at 3.33 seconds. This means that it is not only slower but also less predictable than the other models. These results also allow us to appreciate the improvements of Claude 3.7 Sonnet over Claude 3 Sonnet. The average latency of Claude 3.7 Sonnet is 13.94 seconds per query, which is a significant improvement over Claude 3 Sonnet. However, it is still slower than the other models. The standard deviation of Claude 3.7 Sonnet is also higher than that of Claude 3 Haiku, which means that it is less predictable, for our use case at least.
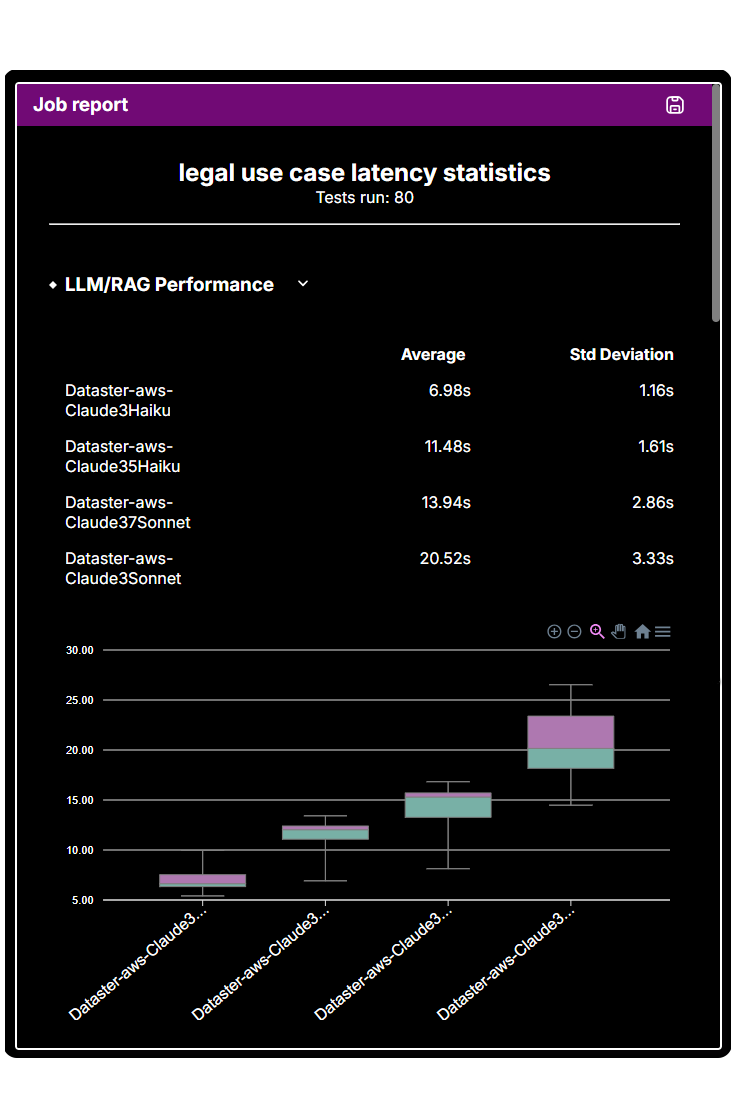
Cost
The Latency Test feature also puts the relative latency of the models in perspective of the cost of using them. Dataster meters actual input and output tokens for your actual use case and uses public pricing to calculate a normalized relative cost index. Similarly, the latency test results are normalized to a relative latency index. The results are then combined to give a relative cost index that takes into account both latency and cost. The results are shown on the graph below. They clearly indicate that Claude 3 Haiku is the best model for our use case thus far as it shows the lowest latency as well as the lowest cost. Claude 3.5 Haiku is the next best option, followed by Claude 3.7 Sonnet and finally Claude 3 Sonnet, which is the most expensive model with the highest latency.
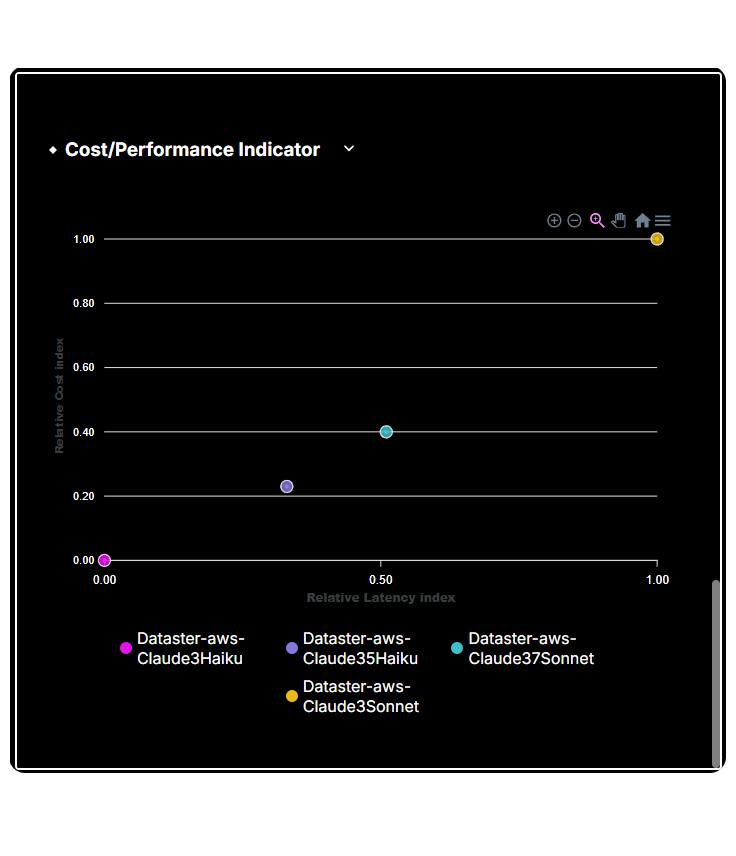
Performance
We want our legal assistant to be accurate and reliable. Dataster supports both a Human Evaluation and an Auto Evaluation feature. The Auto Evaluation feature is particularly useful when you do not have the industry-specific knowledge to judge the quality of answers in-house or when the size of the use case is such that having a human rate all the answers becomes untractable. We will evaluate the performance of each model using the Auto Eval Performance Test feature. This feature allows us to measure the accuracy of each model in responding to a set of queries. Each response is graded as good or bad and the feature returns the average accuracy across all the queries in the use case as well as the actual counts of good and bad responses. We will run the test on the same set of queries that we used to build the use case file and compare the results. We have 1 system prompt and 20 user prompts in the use case file that we want to systematically run against 4 models. So Dataster will run 80 tests in total.
As it turns out, and perhaps surprisingly, the results of the Auto Eval Performance Test show that Claude 3 Haiku's performance exceeds that of all the other models. It achieved an impressive accuracy of 94%, followed by Claude 3 Sonnet at 88%, Claude 3.5 Haiku at 80%, and finally Claude 3.7 Sonnet at 78%. Anecdotally, we see that the total count of tests taken into account to compute the accuracy is not exactly the 80 that we expected. This is because some of the tests were throttled by our model providers. Those are simply just ignored in the computation. These test results highlight that newer models are not always better for every use case.
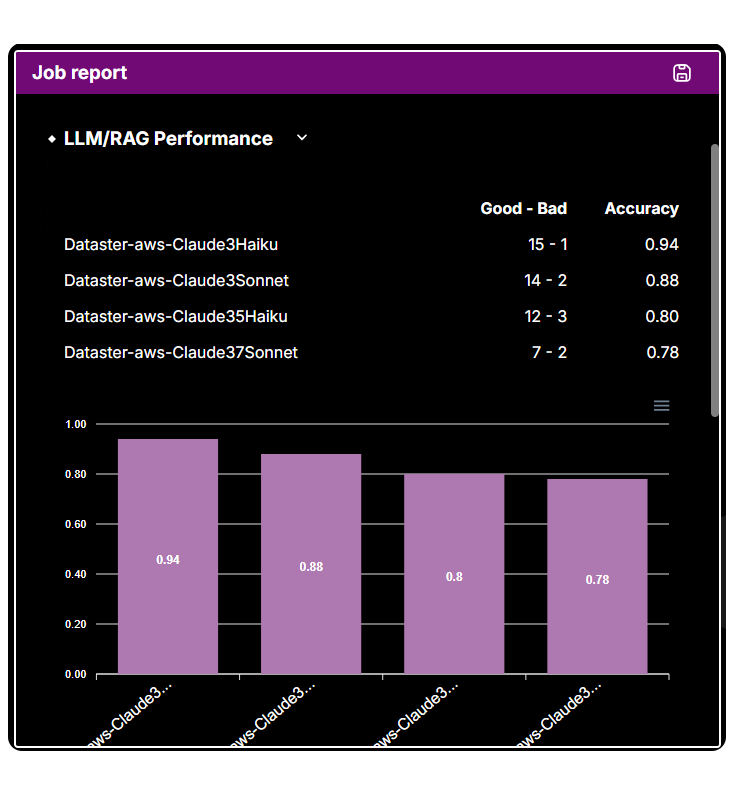
Conclusion
After thorough evaluation, it is clear that Claude 3 Haiku stands out as the best choice for our legal assistant use case. This model excels in several key areas:
- Latency: Claude 3 Haiku is the fastest model, with an average latency of 6.98 seconds per query and the lowest standard deviation of 1.16 seconds. This ensures quick and predictable responses, which is crucial for a responsive legal assistant.
- Cost: Claude 3 Haiku also offers the lowest cost among the models evaluated. The combination of low latency and cost makes it the most economical choice for our use case.
- Performance: The Auto Eval Performance Test reveals that Claude 3 Haiku achieves an impressive accuracy of 94%, outperforming all other models. This high accuracy ensures reliable and relevant responses to complex legal queries.
Public benchmarks can be misleading when selecting an AI model for a specific use case. They typically measure performance across a broad range of tasks, which may not reflect the unique demands of specialized applications like legal tech. For instance, a model that performs well in general language understanding might not excel in understanding complex legal terminology or providing accurate legal advice.
This is where Dataster comes into play. Dataster allows you to create tailored benchmarks that reflect the specific needs of your use case. By using features like the Latency Test and Auto Eval Performance Test, you can measure the performance, cost, and accuracy of different models in the context of your application. This ensures that you choose the model that best meets your requirements, rather than relying on generalized public benchmarks.
Dataster provides a comprehensive platform for evaluating AI models in a way that is directly relevant to your use case. Its features enable you to:
- Measure Latency: Evaluate how quickly models respond to queries, ensuring that your application remains fast and responsive.
- Assess Cost: Calculate the cost of using different models, helping you choose the most economical option.
- Evaluate Performance: Use automated and human evaluation features to measure the accuracy and reliability of models in your specific context.